New Publication Alert: Machine Learning for Predicting Chemical Emission Incidents Triggered by Natural Hazards
- Binbin Lin
- Mar 19
- 1 min read
We are pleased to share that a new study co-authored by Dr. Lei Zou has been published in Process Safety and Environmental Protection:
📄 "Understanding the Effects of Natural Hazards on Chemical Emission Incidents Using Machine Learning Techniques"
Authors: Haoyu Yang, Chi-Yang Li, Lei Zou, and Qingsheng Wang
This research investigates natural hazard-triggered technological accidents (Natechs), focusing on the impact of extreme weather conditions on chemical emission incidents in Houston, TX. The study employs machine learning models—including XGBoost, Random Forest, k-nearest neighbor (kNN), and support vector machine (SVM)—to predict high-risk days for chemical releases based on local climate data.
Key findings include:
1. XGBoost and Random Forest demonstrated the highest predictive performance, with ROC AUC scores exceeding 0.8.
2. Precipitation and lightning were identified as the most influential climate factors contributing to Natechs.
3. The study introduced conformal prediction techniques to optimize the trade-off between sensitivity and specificity, enhancing model reliability.
Co-authors Dr. Lei Zou is a faculty member in the Department of Geography at Texas A&M University, while Haoyu Yang, Chi-Yang Li, and Qingsheng Wang are affiliated with the Artie McFerrin Department of Chemical Engineering at Texas A&M University.
🔗 Read the full paper in PSEP: https://www.sciencedirect.com/science/article/pii/S0957582025001673
📸 Key Figures from the Study:
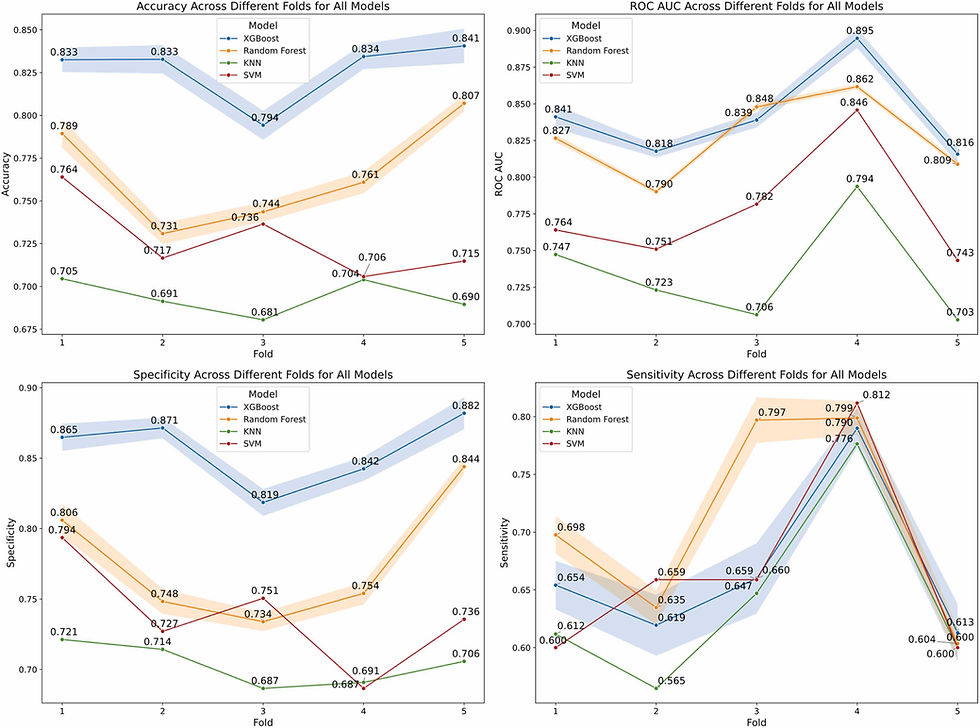
Comments